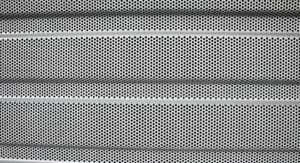
Edge Computing: A Game-Changer for the Internet of Things
Edge Computing: A Game-Changer for the Internet of Things In the era of digital transformation, where the Internet of Things (IoT) has become an integral
Edge Computing: A Game-Changer for the Internet of Things In the era of digital transformation, where the Internet of Things (IoT) has become an integral
Edge computing is a distributed computing model that allows data to be processed closer to the source, whether it be a sensor, device, or user.
San Francisco, CA, March 22, 2024 –(PR.com)– Ngram, a pioneering generative AI company in the life sciences industry, today announced the release of its medchat-qa-descriptive dataset
Los Angeles, CA, March 14, 2024 –(PR.com)– In response to the evolving demands of leadership in our rapidly changing world, Author Ms. Nicky Dare, a renowned
Houston, TX, March 31, 2023 –(PR.com)– The customers need for a growth capital solution required the ability to finance cash payments for inventory being manufactured overseas
Houston, TX, December 30, 2023 –(PR.com)– The customer, a global technologies and materials group, that facilitates nano materials into next generation of commercial technologies and industrial